Personen
Prof. Dr. Martin Weiß
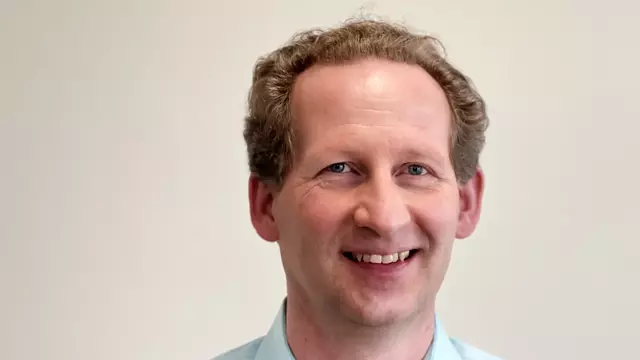
Prodekan
Professor für Numerische Mathematik
Lehre
Lehrgebiete
- Numerische Mathematik
Vorlesungen auf ELO
Abschlussarbeiten
Siehe K:\Wma\Abschlussarbeiten\Abschlussarbeiten.pdf
Forschung
Forschungsinteressen
- Numerische Mathematik
- Robotik
- Maschinelles Lernen
Labor(e)
Forschungseinheit
- Cluster Robotik und Big Data
Werdegang
Studium
- 1990-1995 Mathematik und Informatik, Universität Augsburg
Promotion
- 1999 „Learning Periodic Signals with Recurrent Neural Networks”
Technische Universität Kaiserslautern
Berufliche Erfahrungen
- 1998-1999 wissenschaftlicher Angestellter, Fraunhofer Institut für Techno- und Wirtschaftsmathematik, Kaiserslautern, Abteilung Adaptive Systeme
- 1999-2012 KUKA Robot Group, Augsburg
Entwickler, Projektleiter, Teamleiter Vorentwicklung und Entwicklung
Funktionen intern
- Prodekan Fakultät Informatik und Mathematik
Publikationen/Vorträge
- Weiß, M. G. (2021): Optimization of Cartesian Tasks with Configuration Selection. 2nd IMA Conference on Mathematics of Robotics, Oxford.
- Bock, S.; Weiß, M. G. (2021): Local Convergence of Adaptive Gradient Descent Optimizers. Preprint. OTH Regensburg
- Bock, S.; Weiß, M. G. (2019): Rotation Detection of Components with Convolutional Neural Networks. Preprint. OTH Regensburg
- Bock, S.; Weiß, M. G. (2019): Non-Convergence and Limit Cycles in the Adam optimizer. In: International Conference on Artificial Neural Networks ICANN 2019. München: Springer, 232-243.
- Bock, S.; Weiß, M. G. (2019): A Proof of Local Convergence for the Adam Optimizer. In: International Joint Conference on Neural Networks IJCNN 2019. Budapest, 1--8.
- Weiß, M. G. (2019): Optimal Object Placement Using a Virtual Axis. In: Jadran Lenarcic und Vicenzo Parenti-Castelli: Advances in robot kinematics 2018, Bd. 8. Cham: Springer (Springer Proceedings in Advanced Robotics, 8), S. 116–123.
- Weiß, M. G. (2018): Optimization of Robot Tasks with Cartesian Degrees of Freedom using Virtual Joints. In: 3. OTH-Clusterkonferenz. OTH Regensburg / OTH Amberg-Weiden, S. 75–79.
- Bock, S.; Goppold, J.; Weiß, M. G. (2018): An improvement of the convergence proof of the ADAM-Optimizer. In: 3. OTH-Clusterkonferenz. OTH Regensburg / OTH Amberg-Weiden, S. 80–84.
- Volbert, K.; Weiß, M. G. (2017): Intelligente Steuerung von Industrierobotern. In: 2. OTH-Clusterkonferenz. OTH Regensburg / OTH Amberg-Weiden, S. 16–19.
- Bock, S.; Weiß, M. G. (2017): Lösung der Rückwärtskinematik mit Homotopie-Methoden. In: 2. OTH-Clusterkonferenz. OTH Regensburg / OTH Amberg-Weiden, S. 24–27.
- Weiß, M. G. (2015): A Class of 6R Robots and Poses with 16 Analytical Solutions. In: Proceedings of the IMA Conference on Mathematics of Robotics. IMA Conference on Mathematics of Robotics, 9th September 2015: Institute of Mathematics and its Applications.
- Kurze, M.; Weiß, M.; Otter, M. (2006): Methods and tools to design and test robot control systems. In: Proceedings of the Joint Conference on Robotics: ISR 2006, 37th International Symposium on Robotics and Robotik 2006, 4th German Conference on Robotics; Düsseldorf: VDI-Wissensforum-IWB-GmbH (VDI-Berichte), S. 271–278.
- Townley, S.; Ilchmann, A.; Weiss, M. G.; McClements, W.; Ruiz, A. C.; Owens, D. H.; Prätzel-Wolters, D. (2000): Existence and learning of oscillations in recurrent neural networks. In: IEEE Trans. Neural Netw. 11 (1), S. 205–214. DOI: 10.1109/72.822523 .
- Weiß, M. G. (1998): Learning Periodic Signals with Discrete Time Neural Networks. In: Alessandro Beghi (Hg.): Proceedings of the MTNS-98 Symposium: Mathematical Theory of Networks and Systems. Padova: S. 961–964.
- Weiß, M. G. (1998): Learning periodic signals with recurrent neural networks. In: Z. angew. Math. Mech. 78 (S3), S. 1131–1132. DOI: 10.1002/zamm.199807815130 .
- Weiß, M. G. (1999): Regulation Thermography and Long-Term ECGs: Mathematical Diagnosis Aiding in Medicine. In: Proceedings of the International Congress on Industrial and Applied Mathematics ICIAM 99, Edinburgh. Oxford, New York: Oxford University Press (VDI-Berichte), S. 147.
- Weiß, M. G. (1999): Learning periodic signals with recurrent neural networks. Zugl.: Kaiserslautern, Univ., Diss., 1999. Aachen: Shaker (Berichte aus der Mathematik).
- Weiß, M. G. (1997): Learning oscillations using adaptive control. In: Wulfram Gerstner, Alain Germond, M. Hasler und Jean-Daniel Nicoud (Hg.): Artificial Neural Networks ‐- ICANN’97. Berlin, Heidelberg: Springer Berlin Heidelberg, S. 331–336.